Team Members: Dr.P.Subashini, CMLI Coordinator, Professor of Computer Science
Dr.R.Janani, Research Assistant, CMLI
Ms.Logavathani R , II MCA , Department of Computer Science
Project Summary:The primary goal of this project is to detect and classify seizures and other types of harmful brain activity using EEG signals recorded from the critically ill patients. The significance of the work is to improve electroencephalography pattern classification accuracy which helps to unlock transformative benefits for neurocritical care, epilepsy and drug development. The classification is done in the six patterns such as seizure, generalized periodic discharges, Lateralized periodic discharges, Lateralized Rhythmic Delta activity, generalized rhythmic Delta Activity and others. Advancement in this area helps doctors and brain researchers to detect seizures or other brain damage which provide faster and more accurate treatment to the ill patient. The methodology involves loading EEG data recorded from ill patients, preprocessing it to enhance the quality, visualizing to identify patterns, training deep learning models like EfficientNetV2 and others, on labeled data and using these models to classify EEG signals into patterns like seizures, aiding in neurocritical care, epilepsy treatment, and drug development advancements. Finally, it helps to predict the patterns such as seizure, generalized periodic discharges, Lateralized periodic discharges, Lateralized Rhythmic Delta activity, generalized rhythmic Delta Activity and others. This project employs various deep learning algorithms such as EfficientNetV2, DenseNet, ResNet, and MobileNet, for classifying seizures and other brain activities based on EEG signals. Among these EfficientNetV2 demonstrates exceptional efficiency in pattern classification contributing to the project's advancements in neurocritical care, epilepsy treatment and drug development.
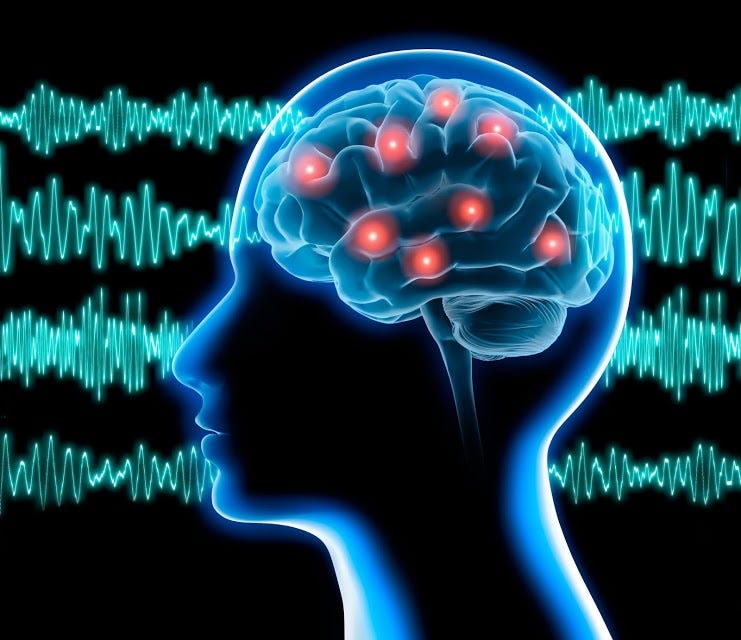